Clinical Data Validation: Ensuring Accuracy and Quality in Clinical Trials
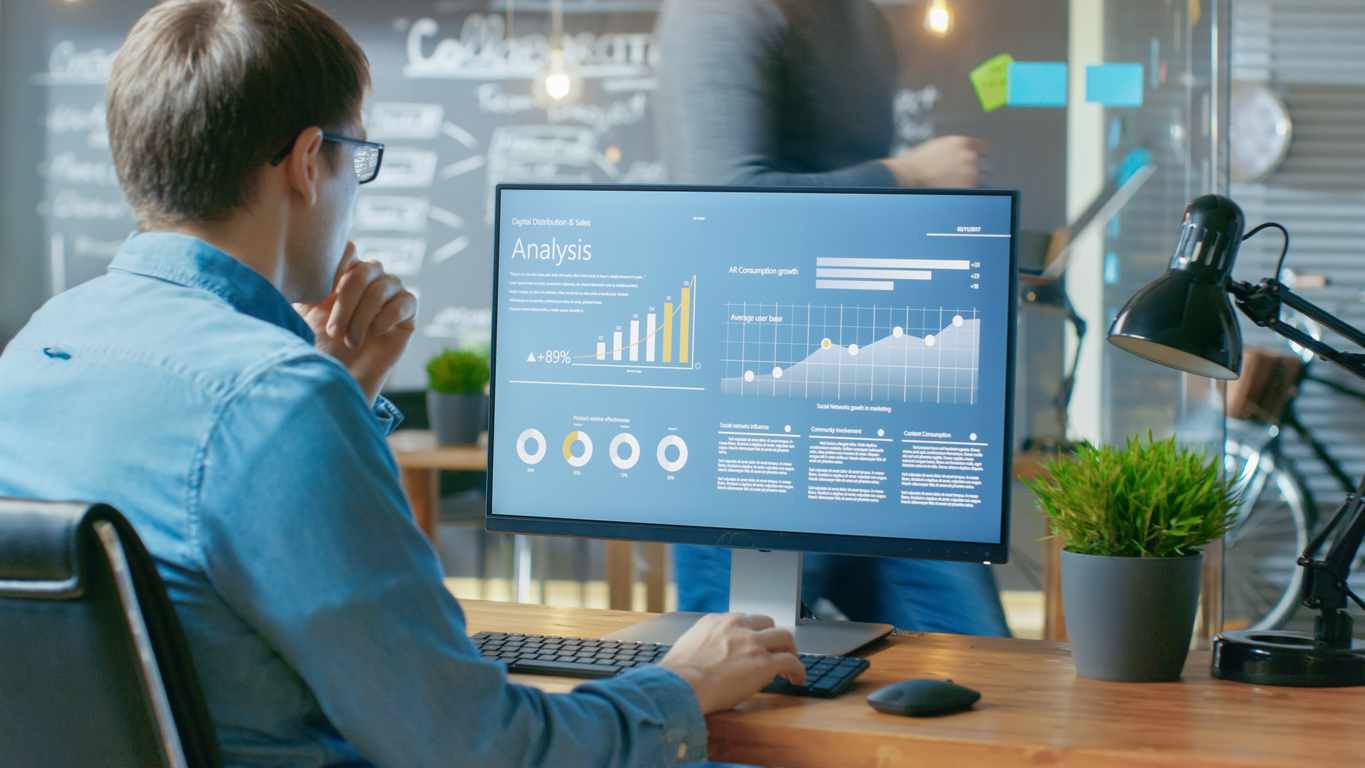
Clinical trials are an essential part of medical research, providing valuable insights into the effectiveness and safety of new treatments.
However, the success of these trials heavily relies on the accuracy and quality of the data collected.
To ensure the validity of the data being collected, robust mechanisms for data validation need to be in place.
In this guide to clinical data validation, we will explore the importance of data validation in clinical studies and the processes involved.
We will also discuss the tools and standards used in clinical data management and provide tips on setting up effective edit checks to prevent data entry errors.
Importance of Data Validation in Clinical Studies
Data validation is fundamental in clinical studies as it ensures that the collected data is reliable, accurate, and suitable for analysis.
Valid data is essential for trial sponsors, researchers, and medical professionals to make informed decisions about patient care and treatment plans.
Inaccurate or incomplete data can have detrimental effects on patient health and the overall population.
Validating data in clinical studies is particularly important because it helps evaluate the worth of research and the ultimate product.
It also ensures compliance with rules and regulations set by regulatory authorities.
Research institutes and pharmaceutical companies invest heavily in data validation techniques to maintain data integrity and meet regulatory requirements.
Overview of Clinical Data Validation
Clinical data validation involves a series of processes to ensure the quality and integrity of clinical data.
These processes are carried out to ensure that the data is attributable, legible, contemporaneous, and original.
Attributability refers to clear documentation of the data source, ensuring that the data can be traced back to its origin.
Legibility ensures that the data is readable and understandable.
Contemporaneousness ensures that the data is recorded in real-time, accurately reflecting the events as they occur.
Lastly, originality ensures that the data is not copied or duplicated from other sources.
If the data fulfills these criteria, it is considered valid and can be used for analysis and decision-making.
Data validation is an ongoing process throughout the clinical trial, starting from the planning stage and continuing until the database is locked.
The Data Validation Process
The data validation process in clinical studies typically involves four main steps: planning, implementation, data checks, and locking the system.
- Planning
During the planning phase, the clinical trial organizers decide on the techniques and procedures to be used for data validation.
This includes documenting the checks, processes, and codes to be implemented.
The planning phase lays the foundation for data validation and ensures that all necessary measures are in place to maintain data integrity.
- Implementation
Once the planning phase is complete, the planned procedures and tests are implemented within the organization’s clinical database system.
This involves setting up the necessary infrastructure, software, and ensuring compliance with regulatory requirements.
The implementation phase ensures that the system is ready to collect and store data accurately.
- Data Checks
After the implementation phase, data checks are performed to ensure the validity of the inputted data.
These checks compare the inputted values against predefined criteria set by the form builder.
The criteria may include numerical limits, logical conditions, or a combination of both. If the inputted data violates any part of the criteria, a warning or query is generated, highlighting the issue.
Invalid results are either fixed or discarded to maintain data accuracy.
- Locking the System
Once the data checks are completed, and all discrepancies are resolved, the system is locked to prevent further amendments without official approval.
Locking the system ensures the integrity of the data and prevents unauthorized changes.
However, it is important to note that a validated system will never delete or modify submitted data, even if it violates the data validation checks.
Instead, the system appends queries to the invalid data, which must be resolved before finalizing the database.
Electronic Data Capture (EDC) Systems for Clinical Data Management
To facilitate data validation and ensure data quality, various software tools are available for clinical data management.
These tools, known as Electronic Data Capture (EDC) systems, provide a platform for data collection, cleaning, and management.
EDC software helps automate data validation processes, maintain an audit trail, and provide easy identification and resolution of data discrepancies.
EDC tools also ensure compliance with regulatory requirements, such as the Code of Federal Regulations (CFR), 21 CFR Part 11.
This regulation governs the use of electronic records in clinical research and requires the use of validated systems to ensure data accuracy, reliability, and consistency.
Edit Checks: Preventing Data Entry Errors
Edit checks are an essential component of data validation in clinical trials.
These checks compare inputted data against predefined criteria to identify discrepancies and errors.
By implementing effective edit checks, data managers can prevent data entry errors and ensure the accuracy and quality of collected data.
Edit checks can be classified into different categories based on their characteristics and effects.
Let’s explore some key types of edit checks:
- Hard vs. Soft Edit Checks
Hard edit checks prevent the user from proceeding to the next item or item group if the inputted data violates any predefined criteria.
It acts as a stop and reassess mechanism, ensuring that only valid data is entered.
Soft edit checks, on the other hand, allow users to continue through the form but prevent them from marking the form complete until the discrepancies are resolved.
Both hard and soft edit checks have their place in clinical data validation.
Hard edit checks are useful for preventing further study activities when an out-of-range value would compromise the study’s integrity or pose safety concerns.
Soft edit checks are more suitable for cases where invalid data is likely due to a typo or misunderstanding, allowing users to proceed while still flagging the issue for resolution.
- Standard vs. Protocol-Specific Edit Checks
Standard edit checks are generic checks that can be applied across different studies.
These checks have reusability and consistency across various clinical trials.
For example, setting a range for body temperature within a vital signs form is a standard edit check that remains consistent across studies.
Standard edit checks are useful for routine measurements and parameters that do not vary significantly between studies.
Protocol-specific edit checks, on the other hand, are tailored to the specific requirements of a particular study protocol.
These checks enforce limits or thresholds dictated by the protocol itself.
- Query Resolution
When data fails to meet the predefined criteria set by edit checks, queries are generated.
Queries serve as red flags, indicating that the data requires attention or clarification.
There are two types of queries: auto-queries and manual queries.
Auto-queries are generated automatically by the system when invalid data is entered.
These queries highlight the discrepancies and must be resolved before the database can be considered locked.
Manual queries, on the other hand, are initiated by human users who have contextual knowledge and can provide additional insights.
Manual queries involve a back-and-forth communication between the data manager and the user to resolve the discrepancy.
Tips for Effective Data Validation
To ensure effective data validation in clinical trials, here are some tips to consider:
Prioritize Safety and Critical Parameters
Place extra scrutiny on values that may signal safety concerns or are critical for the study’s objectives.
Parameters like adverse events, eligibility criteria, and primary endpoints should receive thorough validation.
Consider Context and Study-specific Requirements
Tailor edit checks to the specific needs of the study protocol.
Protocol-specific edit checks ensure that data aligns with the study objectives and endpoints.
Take into account the context and characteristics of the study population to set appropriate ranges and constraints.
Collaborate with Clinical Sites
Work closely with clinical sites to address data discrepancies and resolve queries promptly.
Effective communication and collaboration between data managers and site coordinators are essential for maintaining data accuracy and resolving any issues in a timely manner.
Regularly Review and Update Edit Checks
As the study progresses, review and update edit checks to reflect any changes in the protocol or requirements.
Regularly reviewing and updating edit checks ensures that they remain relevant and effective throughout the study.
Conclusion
Data validation is a key aspect of clinical trials to ensure the accuracy and quality of collected data.
Valid data is essential for making informed decisions about patient care, treatment plans, and regulatory compliance.
By implementing robust data validation processes, utilizing EDC systems, and setting up effective edit checks, researchers can minimize data entry errors and maintain data integrity throughout the trial.
Effective data validation ultimately leads to reliable results and contributes to the advancement of medical knowledge and patient care.
Share This Post
More To Explore
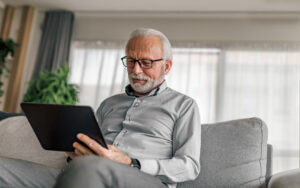
The Evolution of Electronic Patient-Reported Outcomes (ePRO) and Data Collection
If you need an ePRO solution, please contact us at info@klindat.com In the realm of clinical trials and patient registries, the use of Electronic Patient-Reported
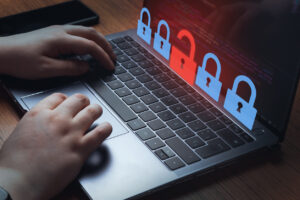
Effective Clinical Database Locks: Ensuring Data Integrity and Reliability
If you need clinical data management services, please contact us at info@klindat.com The integrity and reliability of the data collected in clinical trials are of